A new motion forecasting framework developed by the University of Surrey and Fudan University, China, promises to make autonomous cars both safer and smarter.
Researchers have combined their expertise to create RealMotion – a novel training system that seamlessly integrates historical and real-time scene data with contextual and time-based information, paving the way for more efficient and reliable autonomous vehicle technology.
Existing motion forecasting methods typically process each driving scene independently, overlooking the interconnected nature of past and present contexts in continuous driving scenarios. This limitation hinders the ability to accurately predict the behaviours of surrounding vehicles, pedestrians and other agents in ever-changing environments.
In contrast, RealMotion creates a clearer understanding of different driving scenes. Integrating past and present data enhances the prediction of future movements, addressing the inherent complexity of forecasting multiple agents’ movements.
“Driverless cars are no longer a futuristic dream. Robotaxis are already operating in parts of the USA and China, and self-driving vehicles are expected to be on UK roads as early as next year. However, the real question on everyone’s mind is: how safe are they?,” asks Dr Xiatian Zhu, senior lecturer at the Centre for Vision, Speech and Signal Processing and the Insitute for People-CentredAI at the University of Surrey and co-author of the study. “While AI operates differently from human drivers, there are still challenges to overcome. That’s why we developed RealMotion – to equip the algorithm with not only real-time data but also the ability to integrate historical context in space and time, enabling more accurate and reliable decision-making for safer autonomous navigation.”
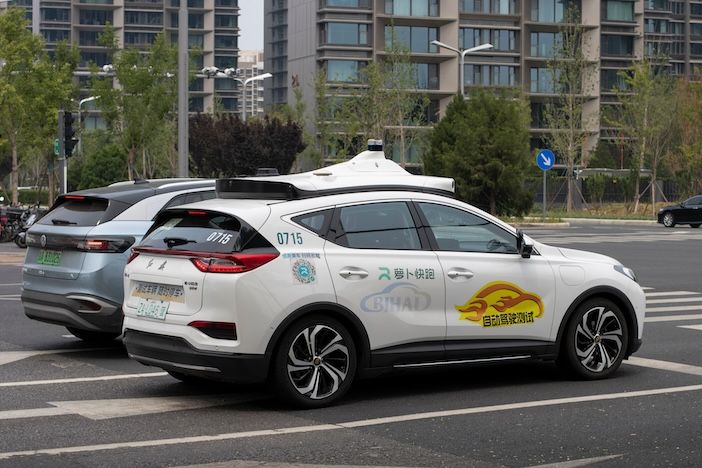
Extensive experiments conducted using the Argoverse dataset, a leading benchmark in autonomous driving research, highlight RealMotion’s accuracy and performance. Compared to other AI models, the framework achieved an 8.60% improvement in Final Displacement Error (FDE) – which is the distance between the predicted final destination and the true final destination. It also demonstrated significant reductions in computational latency, making it highly suitable for real-time applications.
“By equipping autonomous vehicles to perceive their surroundings in real-time, and also leverage historical context to make informed decisions, RealMotion paves the way for safer and more intelligent navigation of our roads,” says Professor Adrian Hilton, director of the Surrey Institute for People-Centred AI.
While researchers encountered some limitations, the team plans to continue its research to further improve RealMotion’s capabilities and overcome any challenges. The framework has the potential to play a critical role in shaping the next generation of autonomous vehicles, ensuring safer and more intelligent navigation systems for the future.